Unveiling: Data Consolidation In Healthcare + More! Discover Now
Ever felt overwhelmed by the sheer volume of disparate data swirling around in today's interconnected world? Data consolidation is no longer a luxury but an absolute necessity for organizations striving for efficiency, insight, and competitive advantage.
The ability to bring together information from various sources into a unified repository unlocks a myriad of possibilities. Imagine a world where data silos crumble, replaced by seamless flows of information that empower informed decision-making at every level. This is the promise of data consolidation, a strategic imperative that is transforming industries and reshaping the future of business. Consider, for example, the transformative impact of data consolidation in healthcare. By aggregating patient data from numerous systems electronic health records, laboratory results, imaging archives, and more healthcare providers can achieve a holistic view of each patient's medical history. This, in turn, enables better care coordination, reduces the risk of medical errors, and ultimately improves patient outcomes. The benefits extend far beyond healthcare, touching every sector from finance and manufacturing to retail and government.
Aspect | Details |
Definition | Data consolidation involves integrating data from multiple sources into a single, unified repository. This can involve data extraction, transformation, cleaning, and loading (ETL) processes. |
Benefits | Improved decision-making, enhanced data quality, increased efficiency, reduced costs, better compliance, and a holistic view of the business. |
Challenges | Data integration complexity, data quality issues, security concerns, scalability requirements, and the need for robust data governance. |
Methods | ETL processes, data virtualization, data warehousing, data lakes, and cloud-based data integration solutions. |
Tools | Informatica PowerCenter, IBM DataStage, Oracle Data Integrator, Talend, and cloud-based platforms like AWS Glue and Azure Data Factory. |
Industries | Healthcare, finance, manufacturing, retail, government, and many others. |
Reference | Gartner Data Consolidation Definition |
Data consolidation is not merely about technical integration; it also entails strategic alignment. Organizations must carefully consider their business goals, data governance policies, and security requirements when implementing a data consolidation strategy. A well-defined roadmap is essential, outlining the specific data sources to be integrated, the transformation rules to be applied, and the desired outcomes to be achieved. Moreover, data quality must be a paramount concern. Inconsistent or inaccurate data can undermine the entire consolidation effort, leading to flawed insights and poor decision-making. Therefore, robust data cleansing and validation processes are crucial to ensure the integrity and reliability of the consolidated data.
- Unleash Your Inner Goddess A Guide To Sexy Lingerie More
- Hyungry Temporary Replacement 2 What Fans Are Saying More
Consider the realm of financial services. Banks and investment firms grapple with massive volumes of data from diverse sources, including customer accounts, trading platforms, risk management systems, and regulatory filings. Consolidating this data into a unified view enables these organizations to gain a deeper understanding of their customers, identify emerging risks, and optimize their business operations. For example, by consolidating customer data from various touchpoints, a bank can develop a more personalized and targeted marketing strategy, resulting in higher customer engagement and increased revenue. Similarly, by consolidating risk data from different systems, a financial institution can improve its ability to detect and mitigate potential threats, safeguarding its assets and protecting its reputation.
The benefits of data consolidation extend far beyond individual organizations. At a societal level, it can facilitate evidence-based policymaking, improve public health outcomes, and promote economic development. For instance, governments can consolidate data from various agencies to gain a more comprehensive understanding of social trends, economic conditions, and environmental challenges. This, in turn, enables them to develop more effective policies and programs that address pressing societal needs. In the realm of public health, data consolidation can help to track the spread of infectious diseases, identify at-risk populations, and improve the delivery of healthcare services. By bringing together data from different sources, public health officials can gain a more complete picture of the health landscape, allowing them to make more informed decisions and allocate resources more effectively.
However, the path to successful data consolidation is not without its challenges. One of the most significant hurdles is the complexity of integrating data from disparate systems, each with its own data formats, data models, and access protocols. Overcoming this complexity requires a combination of technical expertise, sophisticated tools, and a well-defined integration strategy. Another challenge is ensuring data quality. Data errors, inconsistencies, and redundancies can compromise the integrity of the consolidated data, leading to inaccurate insights and flawed decision-making. Therefore, organizations must invest in robust data cleansing and validation processes to ensure that the data is accurate, consistent, and reliable. Security is another critical concern. Consolidating data from multiple sources can create new security vulnerabilities, making it essential to implement robust security measures to protect the data from unauthorized access, modification, or disclosure. This includes implementing strong authentication and authorization controls, encrypting sensitive data, and monitoring the system for suspicious activity.
- Christian Kane From Leverage To Paradise His Life Career
- Aagmaal Unveiling The Truth Behind The Trending Web Series
In today's rapidly evolving technological landscape, cloud-based data consolidation solutions are gaining increasing popularity. These solutions offer a number of advantages over traditional on-premises approaches, including scalability, cost-effectiveness, and ease of deployment. Cloud-based platforms provide a flexible and scalable infrastructure that can easily adapt to changing data volumes and business requirements. They also offer a range of pre-built connectors and data integration tools that simplify the process of integrating data from various sources. Moreover, cloud-based solutions typically have lower upfront costs and ongoing maintenance expenses compared to on-premises solutions, making them an attractive option for organizations of all sizes.
Choosing the right data consolidation approach depends on a variety of factors, including the size and complexity of the organization, the nature of the data being consolidated, and the specific business requirements. Some organizations may opt for a centralized approach, where all data is consolidated into a single data warehouse or data lake. This approach offers a number of advantages, including a single source of truth, simplified data management, and improved data governance. However, it can also be more complex and expensive to implement, particularly for organizations with large and complex data environments. Other organizations may prefer a federated approach, where data remains in its original source systems but is accessed through a virtualized layer. This approach offers greater flexibility and agility, as it avoids the need to physically move data. However, it can also be more challenging to ensure data consistency and data quality across different source systems.
Looking ahead, the future of data consolidation is likely to be shaped by several key trends. One trend is the increasing adoption of artificial intelligence (AI) and machine learning (ML) technologies. AI and ML can be used to automate many of the tasks involved in data consolidation, such as data cleansing, data transformation, and data integration. They can also be used to identify patterns and anomalies in the data, providing valuable insights that can be used to improve business decision-making. Another trend is the growing importance of real-time data consolidation. In today's fast-paced business environment, organizations need to be able to access and analyze data in real time to make timely decisions and respond quickly to changing market conditions. This requires a data consolidation infrastructure that can handle high-volume, high-velocity data streams.
The need for data consolidation extends to the realm of scientific research. Consider the complexities of genomic research, where scientists grapple with vast datasets from sequencing experiments, clinical trials, and epidemiological studies. Consolidating this data into a unified platform allows researchers to identify genetic markers associated with specific diseases, develop new diagnostic tools, and personalize treatment strategies. The ability to integrate and analyze these diverse datasets is accelerating the pace of scientific discovery and improving human health. Furthermore, data consolidation plays a pivotal role in environmental monitoring and conservation. By integrating data from satellites, weather stations, and sensor networks, scientists can gain a more comprehensive understanding of climate change, deforestation, and pollution patterns. This information is crucial for developing effective conservation strategies and mitigating the impact of human activities on the environment.
Another compelling application of data consolidation lies in the realm of supply chain management. Businesses rely on intricate networks of suppliers, manufacturers, distributors, and retailers to deliver products to consumers. Consolidating data from these various stakeholders provides end-to-end visibility into the supply chain, enabling businesses to optimize inventory levels, reduce costs, and improve customer service. For example, by integrating data from suppliers, manufacturers can proactively address potential disruptions, such as material shortages or production delays. By integrating data from retailers, manufacturers can gain insights into consumer demand patterns, allowing them to adjust production schedules and optimize inventory levels. This level of visibility and control is essential for maintaining a competitive edge in today's global marketplace.
In the context of smart cities, data consolidation is paramount for creating sustainable, efficient, and livable urban environments. By integrating data from transportation systems, energy grids, and public safety networks, city planners can optimize resource allocation, improve traffic flow, and enhance public safety. For instance, by consolidating data from traffic sensors, cities can implement adaptive traffic management systems that dynamically adjust signal timings to reduce congestion and improve travel times. By integrating data from energy grids, cities can optimize energy consumption, reduce greenhouse gas emissions, and promote the use of renewable energy sources. By integrating data from public safety networks, cities can improve emergency response times, reduce crime rates, and enhance public safety.
The challenges associated with multilingual data add another layer of complexity to the data consolidation process. Organizations operating in global markets often deal with data in various languages, requiring specialized tools and techniques for data translation and normalization. Accurate translation is crucial to avoid misinterpretations and ensure data consistency. Moreover, cultural differences can impact data interpretation, necessitating a nuanced approach to data analysis. For example, sentiment analysis of customer feedback in different languages may require culturally specific algorithms to accurately capture the nuances of human expression.
Furthermore, the rise of edge computing is creating new opportunities and challenges for data consolidation. Edge computing involves processing data closer to the source, reducing latency and improving responsiveness. This is particularly relevant for applications such as autonomous vehicles, industrial automation, and remote healthcare. However, edge computing also introduces new complexities for data consolidation, as data is generated and processed in geographically distributed locations. Organizations must develop strategies for integrating data from edge devices with data in the cloud or on-premises data centers. This may involve using hybrid cloud architectures and edge-to-cloud data pipelines.
Data governance plays a critical role in ensuring the success of data consolidation initiatives. Data governance encompasses the policies, processes, and standards that govern the collection, storage, use, and dissemination of data. A well-defined data governance framework is essential for ensuring data quality, data security, and compliance with regulatory requirements. This includes establishing clear roles and responsibilities for data management, defining data quality metrics, implementing data security controls, and complying with data privacy regulations such as GDPR and CCPA.
The process of data consolidation often involves transforming data from different formats into a common format. This transformation process can be complex and time-consuming, particularly when dealing with unstructured data such as text documents, images, and videos. Natural language processing (NLP) techniques can be used to extract information from unstructured data and convert it into structured data that can be easily analyzed. Similarly, image recognition and video analytics technologies can be used to extract information from visual data. The ability to process and analyze unstructured data is becoming increasingly important as organizations generate more and more data from diverse sources.
The principles of data consolidation can also be applied to knowledge management. Organizations often struggle to capture and share knowledge effectively, resulting in knowledge silos and missed opportunities. By consolidating knowledge from various sources, such as documents, wikis, and expert systems, organizations can create a unified knowledge base that is easily accessible to employees. This can improve employee productivity, reduce training costs, and foster innovation. The use of AI-powered knowledge management systems can further enhance knowledge sharing and discovery.
The ethical considerations surrounding data consolidation are becoming increasingly important. As organizations collect and consolidate more data, they must be mindful of the potential for bias and discrimination. Data can reflect existing societal biases, leading to unfair or discriminatory outcomes if not carefully addressed. Organizations must implement mechanisms to detect and mitigate bias in data and algorithms. This includes ensuring diversity in data collection, using fair and transparent algorithms, and providing mechanisms for redress when bias is detected.
\u1583\u1405\u1528\u14aa\u1671\u15a6\u15a2\u14c2 \u1431\u15a6\u15a4\u1671\u14f4\u1550\u14c2\u1585 \u140a\u1591\u144e\u14a5\u1483 \u140a\u14d0\u14c4\u154b\u153e\u1528\u1405\u1585\u1450\u1585 \u1403\u1550\u1591\u14ef\u1405\u152d\u1673\u140a\u1550\u14a5\u1483
\u4fee\u6539\u9ed8\u8ba4dns \uff0c\u63a8\u8350alidns\u6216114dns 2.\u8bbf\u95ee bashi5.com \u6216 12580.org \u6216 cldq.cc \u8fdb\u5165\u5730\u5740\u5bfc\u822a 3.\u4e0b\u65b9\u4e0b\u8f7d app \uff0c app\u53ef\u4ee5\u96f6\u6743\u9650\u8fd0\u884c \uff0c\u8bf7\u653e\u5fc3\u5b89\u88c5
\u4fee\u6539\u9ed8\u8ba4dns \uff0c\u63a8\u8350alidns\u6216114dns 2.\u8bbf\u95ee bashi5.com \u6216 12580.org \u6216 cldq.cc \u8fdb\u5165\u5730\u5740\u5bfc\u822a 3.\u4e0b\u65b9\u4e0b\u8f7d app \uff0c app\u53ef\u4ee5\u96f6\u6743\u9650\u8fd0\u884c \uff0c\u8bf7\u653e\u5fc3\u5b89\u88c5
We did not find results for:
Check spelling or type a new query.
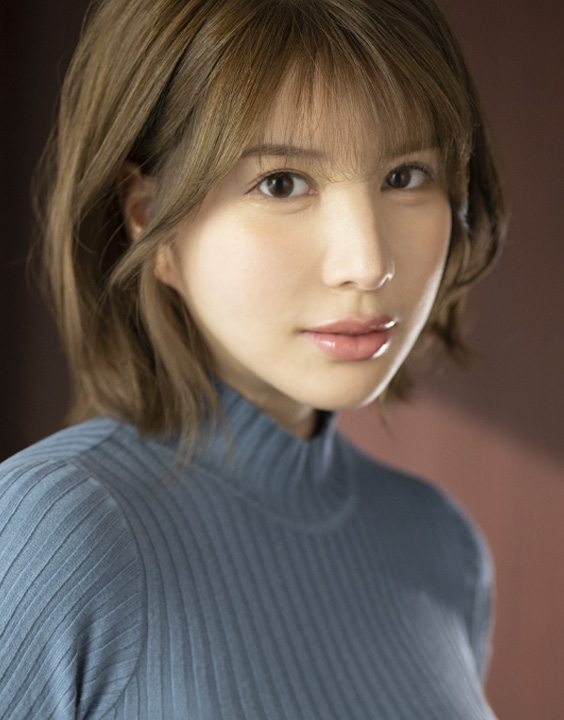
《SSIS 641》凪ひかる2023作品 xb1

凪光(有栖花绯,凪ひかる)2023年新作SSIS 641:为什么突然间改变主意? 尔基
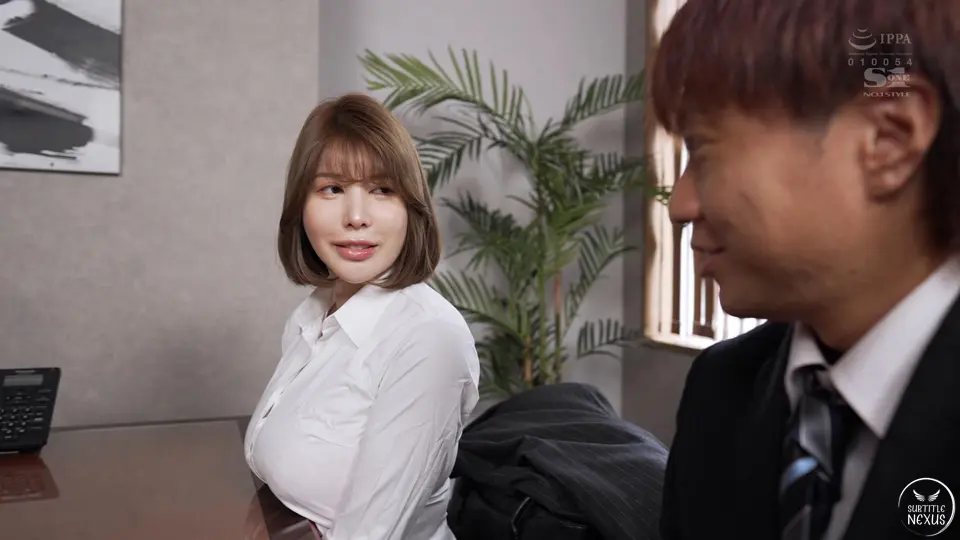
SSIS 641 Subtitles Subtitle Nexus